Creascience’s business analytics unit offers state of the art quantitative tools to implement advanced business analytics procedures. Our mastering of the most recent statistical methods in modelling, data mining & market research coupled with experienced data scientists allows us to help create Business Intelligence for custom problem-solving solutions.
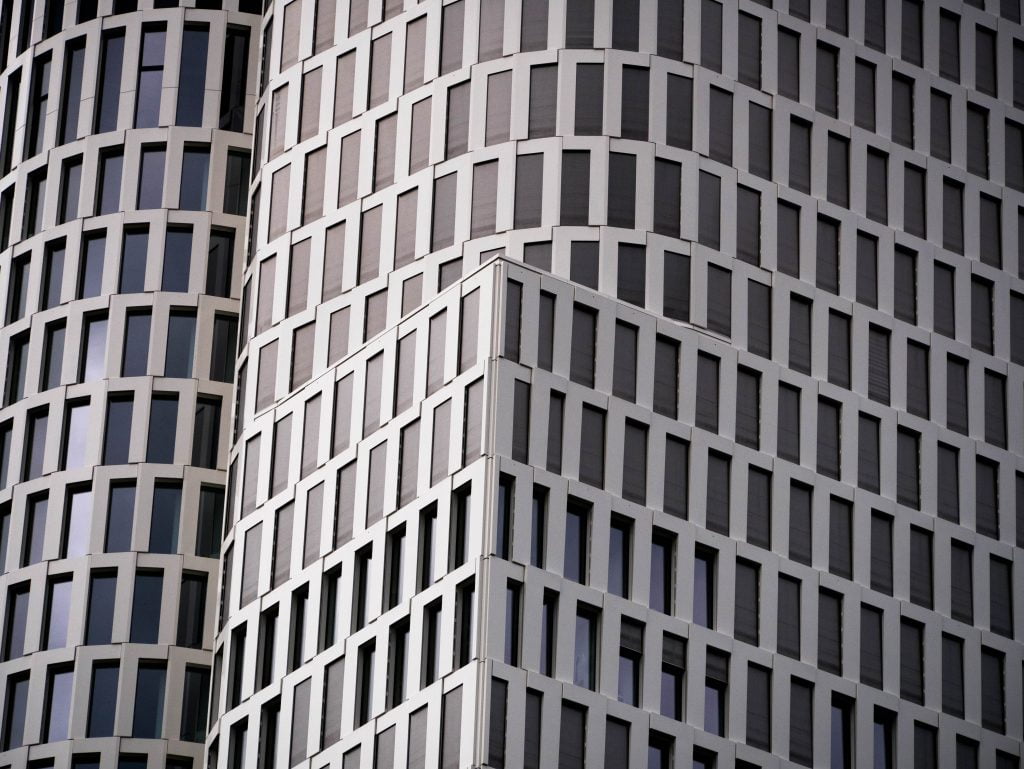
Our area of expertise includes credit scoring, consumer segmentation, HR risk management modelling… We are especially active in the predictive analytics & Big Data – Market Research.
Predictive Analytics & Big Data
Predictive analytics is the science of using existing data sources in order to predict with the best accuracy specific behaviours. It is now used in many disciplines such as financial analysis, auditing and marketing.
Creascience combines its knowledge of these fields of application with an extensive experience in data analysis and modelling to offer tailored solutions especially focused on groups wanting to get started in this field.
MORE
Market Research
It is the wish of all service and product providers to understand and predict consumer behaviour. We offer groundbreaking methods to optimize the quality of your products while integrating consumer needs. Our interventions apply to the design of efficient studies, thorough data analysis and innovative presentation of results.